This post outlines some experiments I ran using Auxiliary Loss Optimization for Hypothesis Augmentation (ALOHA) for DGA domain detection.
(Update 2019-07-18) After getting feedback from one of the ALOHA paper authors, I modified my code to set loss weights for the auxilary targets as they did in their paper (Weights used: main target 1.0, auxilary targets 0.1). I also added 3 word-based/dictionary DGAs. All diagrams and metrics have been updated to reflect this.
I recently read this paper ALOHA: Auxiliary Loss Optimization for Hypothesis Augmentation
by Ethan M. Rudd, Felipe N. Ducau, Cody Wild, Konstantin Berlin, and Richard Harang from Sophos Lab. This research will be presented at USENIX Security 2019 in Aug, 2019. This paper shares findings that supplying more prediction targets to their model at training time, they can improve the prediction performance of the primary prediction target. More specifically, they modify a deep learning based model for detecting malware (binary classifier) to also predict things like individual vendor predictions, malware tags, and number of VT detections. Their “auxiliary loss architecture yields a significant reduction in detection error rate (false negatives) of 42.6% at a false positive rate (FPR) of 10^−3 when compared to a similar model with only one target, and a decrease of 53.8% at 10^−5 FPR.”
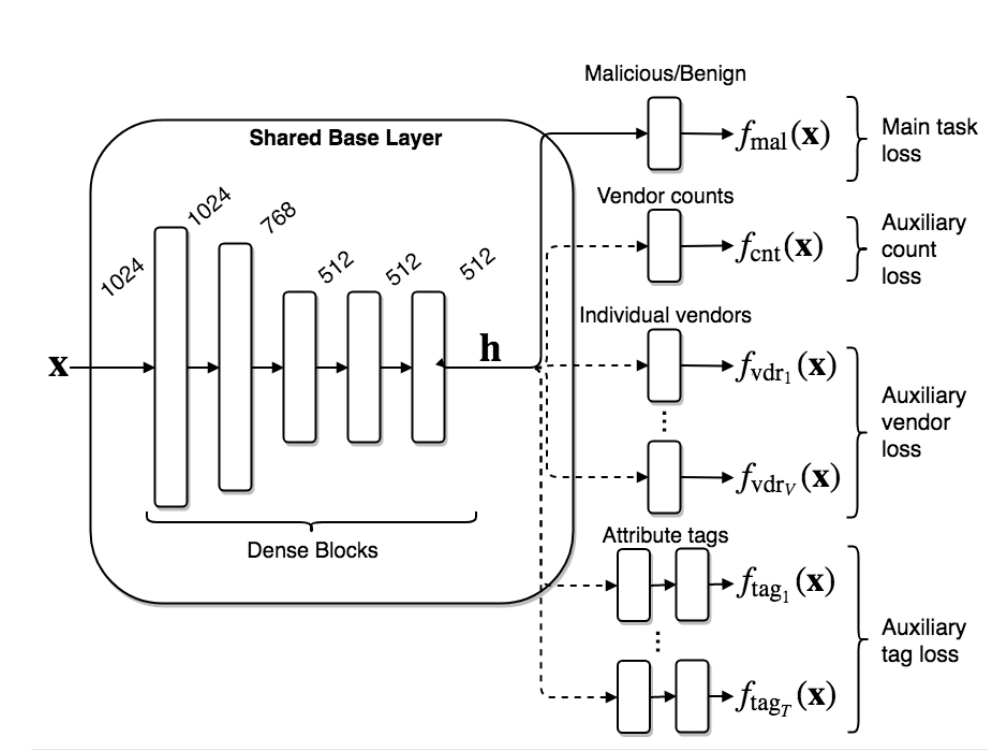
Figure 1 from the paper
A schematic overview of our neural network architecture. Multiple output layers with corresponding loss functions are optionally connected to a common base topology which consists of five dense blocks. Each block is composed of a Dropout, dense and batch normalization layers followed by an exponential linear unit (ELU) activation of sizes 1024, 768, 512, 512, and 512. This base, connected to our main malicious/benign output (solid line in the figure) with a loss on the aggregate label constitutes our baseline architecture. Auxiliary outputs and their respective losses are represented in dashed lines. The auxiliary losses fall into three types: count loss, multi-label vendor loss, and multi-label attribute tag loss
This paper made me wonder how well this technique would work for other areas in network security such as:
- Detecting malicious URLs from Exploit Kits - possible auxiliary labels: Exploit Kit names, Web Proxy Categories, etc.
- Detecting malicious C2 domains - possible auxiliary labels: malware family names, DGA or not, proxy categories.
- Detecting DGA Domains - possible auxiliary labels: malware families, DGA type (wordlist, hex based, alphanumeric, etc).
I decided to explore the last use case of how well auxiliary loss optimizations would improve DGA domain detections. For this work I identified four DGA models and used these as baselines. Then I ran some experiments. All code from these experiments is hosted here. This code is based heavily off of Endgame’s dga_predict, but with many modifications.
Data:
For this work, I used the same data sources selected by Endgame’s dga_predict (but I added 3 additional DGAs: gozi, matsnu, and suppobox).
- Alexa top 1m domains
- classical DGA domains for the following malware families: banjori, corebot, cryptolocker, dircrypt, kraken, lockyv2, pykspa, qakbot, ramdo, ramnit, and simda.
- Word-based/dictionary DGA domains for the following malware families - gozi, matsnu, and suppobox
Baseline Models:
I used 4 baseline binary models + 4 extensions of these model that use Auxiliary Loss Optimization for Hypothesis Augmentation.
Baseline Models:
ALOHA Extended Models (each simply use the 11 malware families as additional binary labels):
- ALOHA CNN
- ALOHA Bigram
- ALOHA LSTM
- ALOHA CNN+LSTM
I trained each of these models using the default settings as provided by dga_predict (except, I added stratified sampling based on the full labels: benign + malware families):
- training splits: 76% training, 4% validation, %20 testing
- all models were trained with a batch size of 128
- The CNN, LSTM, and CNN+LSTM models used up to 25 epochs, while the bigram models used up to 50 epochs.
Below shows counts of how many of each DGA family were used and how many Alexa top 1m domains were included (denoted as “benign”).
1
2
3
4
5
6
7
8
9
10
11
12
13
14
15
16
17
18
19
20
21
22
23
24
| In [1]: import pickle
In [2]: from collections import Counter
In [3]: data = pickle.loads(open('traindata.pkl', 'rb').read())
In [4]: Counter([d[0] for d in data]).most_common(100)
Out[4]:
[('benign', 139935),
('qakbot', 10000),
('dircrypt', 10000),
('pykspa', 10000),
('corebot', 10000),
('kraken', 10000),
('suppobox', 10000),
('gozi', 10000),
('ramnit', 10000),
('matsnu', 10000),
('locky', 9999),
('banjori', 9984),
('simda', 9984),
('ramdo', 9984),
('cryptolocker', 9984)]
|
Results
Model AUC scores (sorted by AUC):
- aloha_bigram 0.9435
- bigram 0.9444
- cnn 0.9817
- aloha_cnn 0.9820
- lstm 0.9944
- aloha_cnn_lstm 0.9947
- aloha_lstm 0.9950
- cnn_lstm 0.9957
Overall, by AUC, the ALOHA technique only seemed to improve the LSTM and CNN models and only marginally. The ROC curves show reductions in the error rates at very low false positive rates (between 10^-5 and 10^-3) which is similar to those gains seen in the ALOHA paper, yet the paper’s gains appeared much larger.
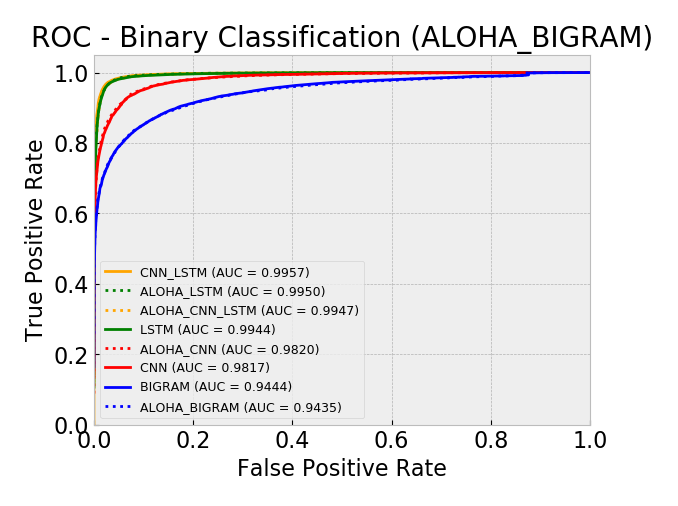
ROC: All Models Linear Scale
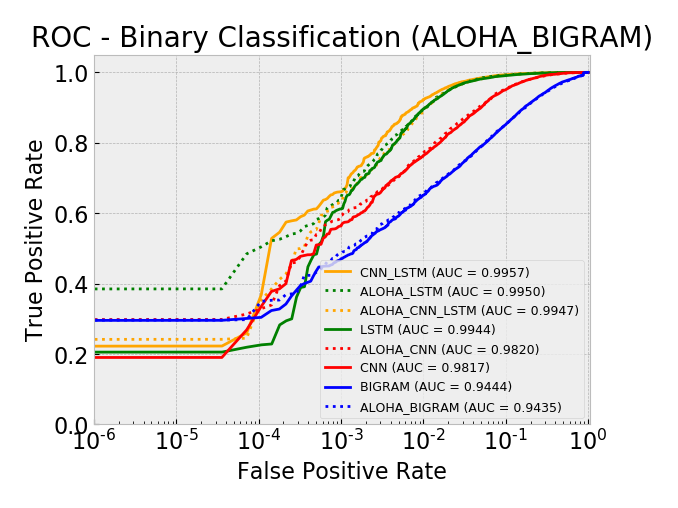
ROC: All Models Log Scale
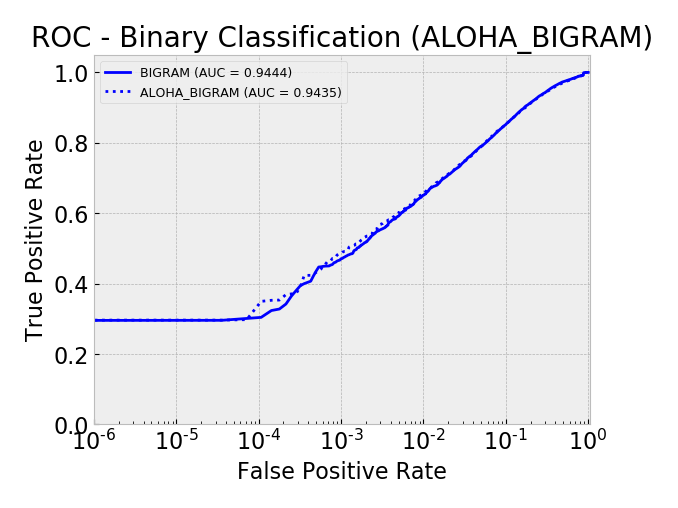
ROC: Bigram Models Log Scale
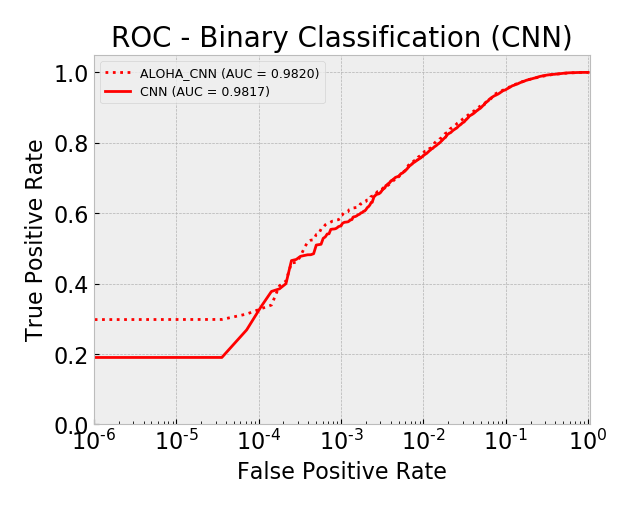
ROC: CNN Models Log Scale
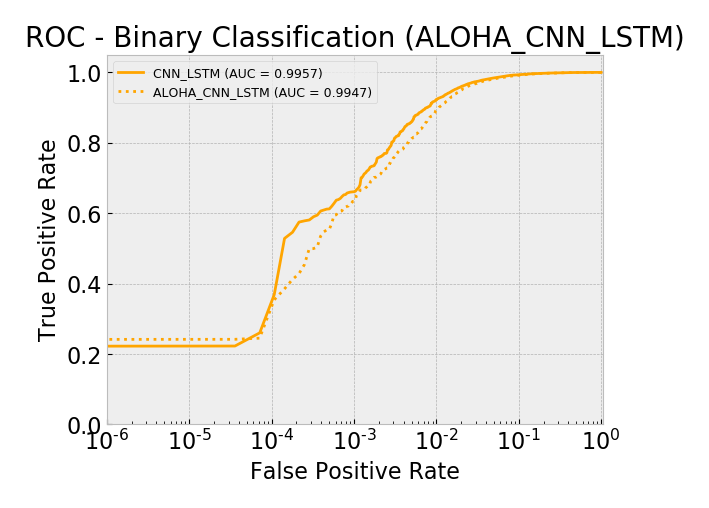
ROC: CNN+LSTM Models Log Scale
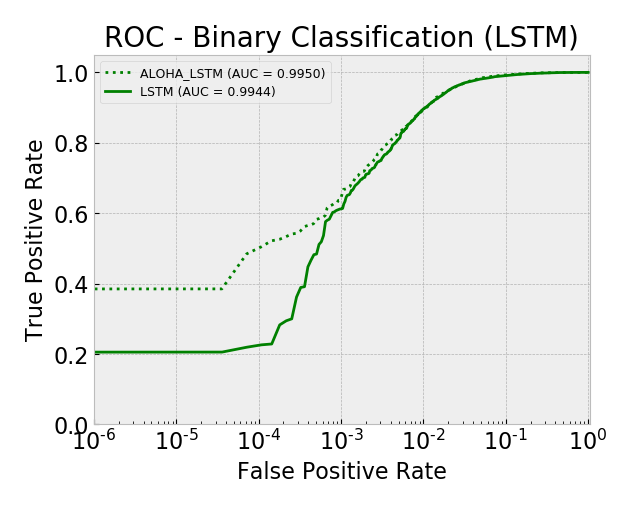
ROC: LSTM Models Log Scale
Heatmap
Below is a heatmap showing the percentage of detections across all the malware families for each model. Low numbers are good for the benign label (top row), high numbers are good for all the others.
Note the last 3 rows are all word-based/dictionary DGAs. It is interesting, although not too surprising that the models that include LSTMs tended to do better against these DGAs.
I annotated with green boxes places where the ALOHA models did better. This seems to be most apparent with the models that include LSTMs and for the word-based/dictionary DGAs.
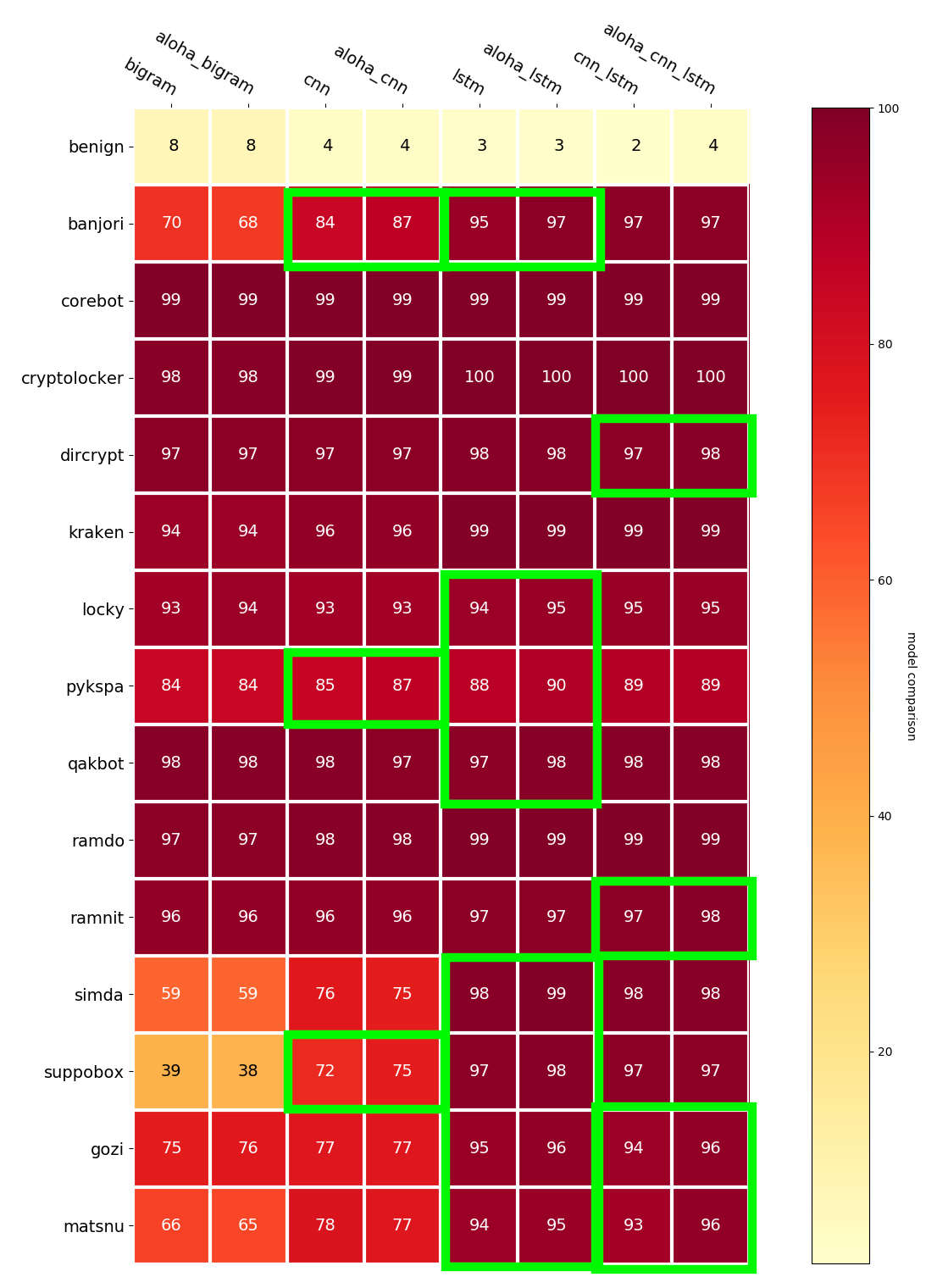
Future Work:
These are some areas of future work I hope to have time to try out.
- Add more DGA generators to the project, esp word-based / dictionary DGAs and see how the models react. I have identified several (see “Word-based / Dictionary-based DGA Resources” from here for more info).
- try incorporating other auxiliary targets like:
- Type of DGA (hex based, alphanumeric, custom alphabet, dictionary/word-based, etc)
- Classical DGA domain features like string entropy, count of longest consecutive consonant string, count of longest consecutive vowel string, etc. I am curious if forcing the NN to learn these would improve its primary scoring mechanism.
- Metadata from VT domain report.
- Summary / stats from Passive DNS (PDNS).
- Features from various aspects of the domain’s whois record.
If you enjoyed this post, you may be interested in my other recent post on Getting Started with DGA Domain Detection Research. Also, please see more Security Data Science blog posts at by personal blog: covert.io.
As always, feedback is welcome so please leave a message here, on Medium, or @ me on twitter!
–Jason
@jason_trost